Dissertations and capstones are significant milestones in the academic journey of students pursuing higher education. These rigorous research projects demand meticulous planning, extensive data collection, and in-depth analysis to derive meaningful conclusions. Among the various phases of a dissertation, data analysis plays a pivotal role in unraveling insights and uncovering trends that contribute to the overall objective of the study. With the aim of understanding the science behind dissertation data analysis, we delve into the intricacies of this critical process and explore the methodologies employed to make sense of the vast pool of information gathered. In this article, we shed light on the essential steps involved in dissertation data analysis, highlighting the strategies that researchers employ to transform raw data into valuable knowledge. So, whether you’re embarking on your own dissertation journey or seeking a deeper understanding of this crucial aspect of academic research, join us in unraveling the science behind dissertation data analysis.
Importance of Dissertation Data Analysis
Data analysis is a crucial aspect of dissertations and capstones, playing a pivotal role in uncovering meaningful insights and contributing to the overall value of research projects. Without effective data analysis, the findings and conclusions drawn from the study may lack credibility and fail to make a significant impact. This section highlights the importance of dissertation data analysis in three key aspects.
- Informing Research Questions and Hypotheses:
Data analysis helps researchers to make informed decisions regarding the direction and focus of their research. By analyzing relevant data, researchers can gain insights into prevailing patterns, trends, and relationships within the dataset. This analysis aids in identifying research gaps, shaping research questions, and refining hypotheses. Furthermore, it enables researchers to select appropriate methodologies and statistical techniques that best fit the data under investigation.
- Ensuring Research Validity and Reliability:
Help With Dissertation Proposal
Validating the findings of a dissertation is of paramount importance in ensuring its credibility and reliability. Through data analysis, researchers can assess the internal and external validity of their study. By analyzing the collected data, researchers can verify the consistency and accuracy of the research instruments, identify any biases, and assess the generalizability of the findings. This rigorous analysis helps to strengthen the overall validity and reliability of the dissertation, making it more robust and trustworthy.
- Drawing Reliable Conclusions and Implications:
The ultimate goal of any dissertation is to contribute to the existing body of knowledge and offer meaningful insights to the field of study. Data analysis plays a vital role in achieving this goal by enabling researchers to draw reliable conclusions and make informed recommendations for future research and practice. By analyzing the collected data, researchers can identify significant patterns, trends, and associations, which can help reinforce or challenge existing theories or assumptions. These findings then form the basis for constructing well-grounded conclusions and outlining practical implications for real-world applications.
In conclusion, dissertation data analysis is an integral part of any research endeavor, offering valuable insights that shape the research process, increase the validity of findings, and provide a foundation for drawing reliable conclusions. Researchers must recognize the importance of dedicating adequate time and expertise to this critical stage of their dissertations to ensure the overall success and impact of their research endeavors.
Key Steps in Dissertation Data Analysis
When embarking on the journey of dissertation data analysis, there are several crucial steps that can guide researchers towards insightful findings. By following a systematic approach, students can effectively analyze the data collected for their dissertations and capstones. This section explores the key steps involved in dissertation data analysis.
Data Preparation: The first step in dissertation data analysis is to ensure that the collected data is organized and ready for analysis. This involves cleaning and coding the data, checking for missing values or outliers, and transforming the data if necessary. By preparing the data meticulously, researchers can ensure the accuracy and reliability of their analysis.
Identifying Research Questions: Before diving into the analysis, it is essential to clearly define the research questions or hypotheses that the data analysis aims to address. This step helps researchers maintain focus and structure their analysis accordingly. By identifying the key research questions, students can select the appropriate statistical methods and techniques for analyzing the data.
Applying Statistical Methods: Once the data is prepared and research questions are defined, the next step is to select and apply suitable statistical methods for analysis. This may involve descriptive statistics to summarize the data, inferential statistics to test hypotheses, or multivariate analysis techniques to explore relationships among variables. Researchers must choose the methods that align with their research goals and ensure the validity and reliability of their analysis.
By following these key steps in dissertation data analysis, students can navigate the complexities of analyzing their research data effectively. The successful analysis of dissertations and capstones demands careful attention to detail, adherence to ethical guidelines, and the utilization of appropriate statistical tools.
Common Challenges in Dissertation Data Analysis
- Limited Sample Size
One of the common challenges faced during dissertation data analysis is the limited sample size. Many times, researchers have to work with a relatively small number of participants or cases, which can affect the generalizability of the findings. With a limited sample size, there is a higher chance of encountering statistical noise or random variations in the data, making it essential for researchers to exercise caution when interpreting the results.
- Data Quality and Integrity
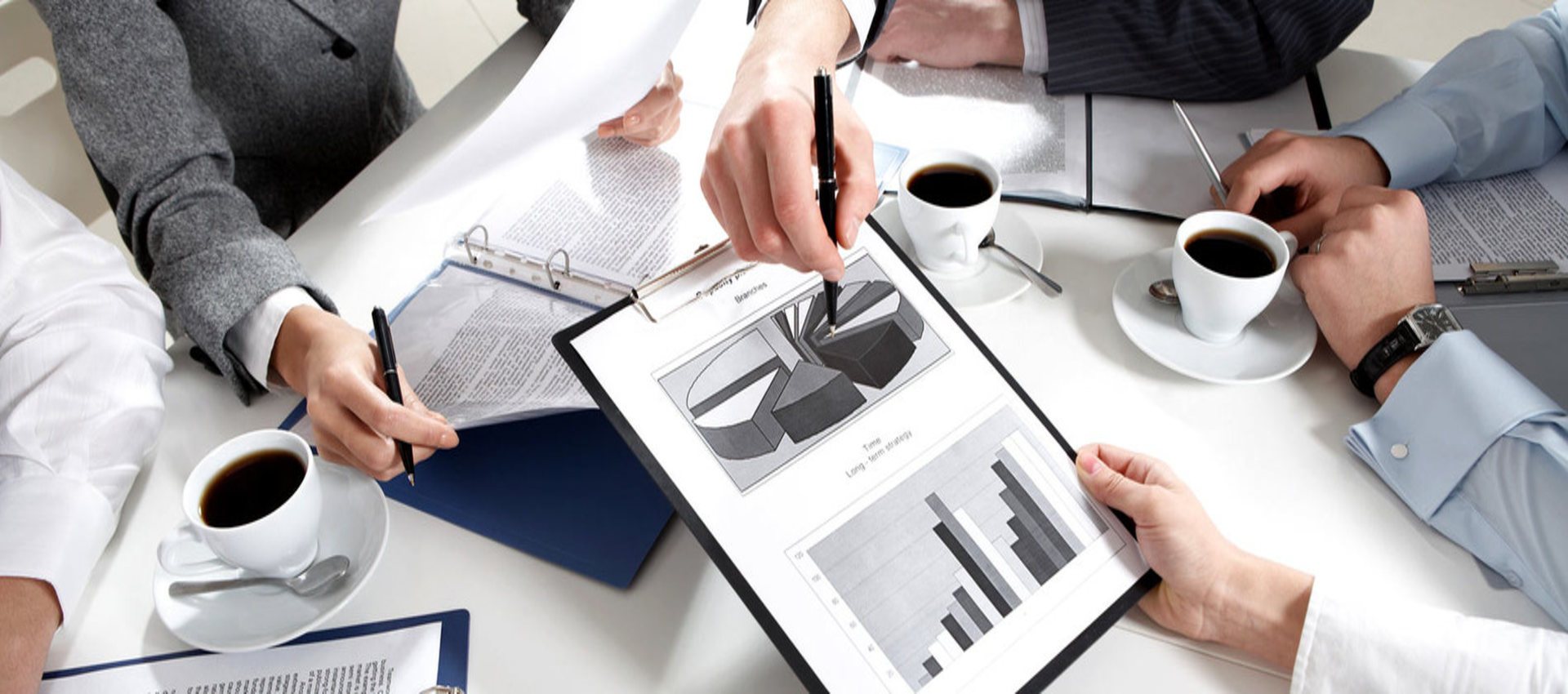
Maintaining data quality and integrity is another significant challenge in dissertation data analysis. Researchers often have to deal with incomplete or inconsistent data sets, missing values, or errors in data entry. Such issues can lead to biases in the analysis and impact the validity and reliability of the findings. It is crucial for researchers to carefully assess the quality of the data and take appropriate measures to minimize any potential errors or biases.
- Data Interpretation and Analysis Techniques
Another challenge lies in selecting and applying appropriate data analysis techniques. Different research questions and data types may require different statistical methods or qualitative analysis approaches. Researchers need to carefully consider the nature of their data and choose the most suitable analysis techniques accordingly. This decision-making process can be complex and may require a good understanding of statistical concepts, research objectives, and the limitations of various analysis methods.
In conclusion, dissertation data analysis involves several common challenges that researchers need to address. These challenges include limited sample sizes, data quality and integrity issues, as well as the selection and application of appropriate analysis techniques. By being aware of these challenges, researchers can ensure the validity and reliability of their findings and contribute to the scientific understanding of their chosen field.